Semiparametric Discrete Choice Models for Bundles - Fu Ouyang
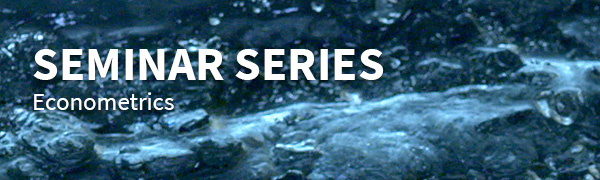
Title: Semiparametric Discrete Choice Models for Bundles
Abstract: We propose two approaches to estimate semiparametric discrete choice models for bundles. Our first approach is a kernel-weighted rank estimator based on a matching-based identification strategy.
We establish its complete asymptotic properties and prove the validity of the nonparametric bootstrap for inference. We then introduce a new multi-index least absolute deviations (LAD) estimator as an alternative, of which the main advantage is its capacity to estimate preference parameters on both alternative- and agent-specific regressors. Both methods can account for arbitrary correlation in disturbances across choices, with the former also allowing for interpersonal heteroskedasticity. We also demonstrate that the identification strategy underlying these procedures can be extended naturally to panel data settings, producing an analogous localized maximum score estimator and a LAD estimator for estimating bundle choice models with fixed effects. We derive the limiting distribution of the former and verify the validity of the numerical bootstrap as an inference tool. All our proposed methods can be applied to general multi-index models. Monte Carlo experiments show that they perform well in finite samples.